元学习:分析样本复杂度
(Meta Learning: Sample Complexity Analysis)
通过前面几篇的背景铺垫,我们现在可以探讨一个具体的学习问题——小样本学习。重温一下样本复杂度:机器学习算法的样本复杂度表示成功学习目标函数所需的训练样本数。样本复杂度线性地取决于目标函数类别上的VC维。
归纳偏置 (Inductive bias)
这个概念是为了描述泛化能力,归纳是inductive learning里的归纳(数学归纳法,推广、泛化),bias是bias-variance tradeoff里的bias。
小样本学习
通过经验风险最小化(ERM)学得 f : X → Y

ER损失函数为
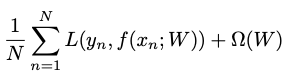
元学习(Meta Learning)
元学习的样本复杂度
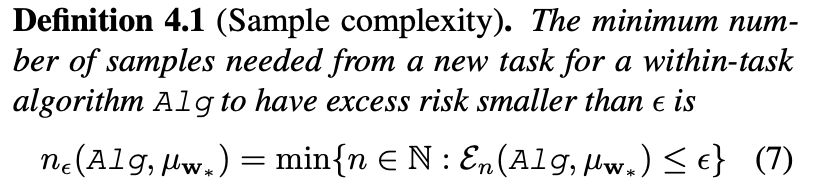
Comentários